A team of engineers from Google Deepmind (creators of AlphaGo) have published a challenge for the AI developer community. The goal is to create Artificial Intelligence capable of reaching the highest scores in a card game called Hanabi.
Table games have always been one of the major study fields of
Artificial Intelligence, as they often require skills that closely match human
intelligence, such as problem resolution, planning or creative thinking, but
with the advantage of being scenarios controlled with a set of defined rules.
In recent years we have seen major advances in this field with programs capable of beating the best human
players in some of the more complex games.
The first was IBM's DeepBlue, which beat the grandmaster Gary
Kasparov. Other examples are DeepStack and Libratus in the poker world, Watson
in the TV game Jeopardy or more recently AlphaGo, from Deepmind, against the
grandmasters of Go.
The question therefore is, having developed AI capable of beating a world champion at a complex game such as Go, why develop now a system to play this little card game?
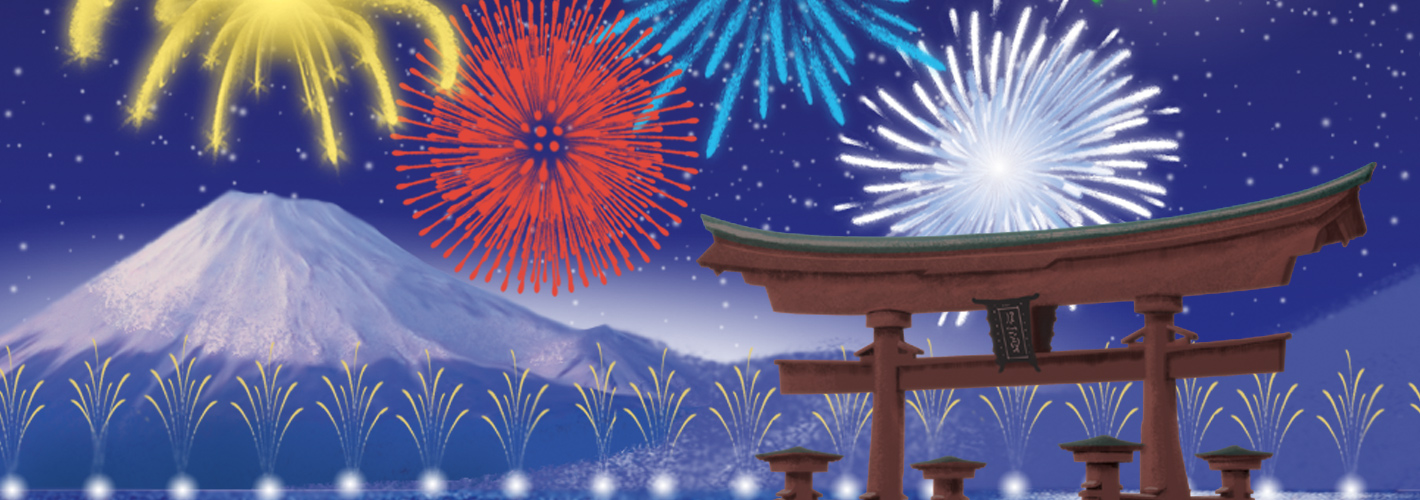
But, what
is Hanabi?Hanabi is a small card
game published in 2010. Contrary to classic games, it is a cooperative game, which means
that all players work together to reach a common goal. In a cooperative game,
either all players win or all lose.
In Hanabi, players try to create "the most spectacular
fireworks show possible". This means they try to play cards of multiple
colours numbered from 1 to 5 in sequence and in their corresponding colour.
The fun of the game is that you play with the cards placed upside
down, so that each player is unaware of the cards in his hand and does not see
the card he is going to play.
Each turn involves doing of the following three actions: discard a
card to get a hint card, spend one of those cards to give a hint, or try to
play a card.
Hints are very limited and consist in telling a player how many
cards he has of a certain colour or number. Some hint examples include
"These two cards have a value of 3" or "these three cards are
white" or "you do not have any 4 in your hand".
When you try to play a card, if it is not valid because it is not a
matching card with those on the table, it is marked as a failure. The game ends
in defeat after 3 failures. If this does not happen, the game ends when the
last card is taken from the deck and the score is calculated.
The score is the sum of the highest card played correctly of each
colour. Therefore, the maximum score is 25 points.
Why Hanabi?
Large Artificial Intelligence companies have already managed to
develop systems that are capable of beating the best human players at much more
complicated games. So why dedicate time and effort to a relatively unknown card
game?
Mainly because Hanabi poses challenges that are different to other games. Chess, Go and many others are "Zero Sum games", which means there is only one winner and others lose.
In addition, most of them are two-player games. Hanabi requires collaboration and communication, which are different
challenges to those raised by the other games, and therefore, skills that
the agents have not developed until now.
To make things more interesting and delve into those two aspects,
the proposed challenge ultimately aims
for the agent to be able to play with humans.
Hanabi is a game based on communication as its main playing
mechanism. This is an enormous challenge for Artificial Intelligence, but the
advantage Hanabi can have over other environments is that communication is
perfectly regulated and limited.
The information that can be transmitted is finite and can only be
communicated at certain times. In addition, paralinguistic communication is excluded, i.e. gestures,
intonations, postures, etc. are not allowed.
In any case**, the need to
communicate means the agent must be capable of establishing an effective
communications protocol with the other players**. To do this, there must be a
way to establish a series of communication rules within the group of players.
Considering it can also play with humans, the agent must be capable of establishing a communications protocol
during the game. The added problem is that, unlike programming agents,
humans tend to adopt these conventions organically, redesigning them during one
or more games, instead of establishing a series of fixed rules and observing
them perfectly.
However, even if the agent is able to establish an effective
communications protocol, what makes this game really interesting is that the
information transmitted is not only limited to the hint itself, but is also influenced
on the when it was given, greatly depending on the intention of each hint
within the context of the game.
For example, if at the start of the game someone tells me I have a
4, which is a card I cannot play; this means I can drop this card without fear
and not the others. If the hint is provided when there is a green 3 on the
table, it probably means the 4 is a green 4 and I should play it.
Therefore, Artificial Intelligence must be capable of inferring the purpose of the actions of the other players. This is where the area of study that in Cognitive Sciences is known as Mind Theory comes into play. This can be summarised into a few words as how to guess what the other is thinking and what are the intentions of their actions.
To add even more difficulty, one cannot make a very common assumption of game resolution in the development of this Artificial Intelligence, known as Nash Equilibrium, whereby AI assumes that all players adopt the best possible strategy.
So, this agent must be capable of deducing the ultimate purpose of
each action, seeking to infer what the other player had in mind, bearing in
mind that the other player may not have chosen the optimal card and that the
communications protocol may be imperfect.
One wonders whether the problem of the Mind Theory was not resolved
as those agents were playing poker. The answer is no, because when assuming the
Nash Equilibrium, players are taken for granted to always choose the best
strategy and in poker this entails providing the least amount of information
possible in each move.
Therefore, AI agents were programmed on the assumption that
opponents do not transmit any additional information. That is, they never tried
to read the opponent's intentions.
The Deepmind team. which has raised the challenge, has already faced
the problem and has realised that the
use of the latest Deep Learning techniques present in the currently most
advanced Artificial Intelligences such as AlphaGo**, are unable to properly resole this game**.
This type of techniques does not perform as well as a simpler
program developed with a set of predefined behaviours in the code. This is what
has drawn the attention of this team and what has led them to launch this
challenge.
It is clear there is still a long way to go to reach the mythological general Artificial Intelligence, but this may be another small step in that direction. For anyone who is encouraged to take part or just curious, the article with the challenge is here and they have also published a test environment in Github.
Sources
Comments are moderated and will only be visible if they add to the discussion in a constructive way. If you disagree with a point, please, be polite.
Tell us what you think.