For some time now, the role of Data Scientist has emerged on the market, understood as the data guru, the expert in the new stream of Data Science… but where did this new position come from? Until they arrived, nobody analyzed data? Nobody took advantage for business?
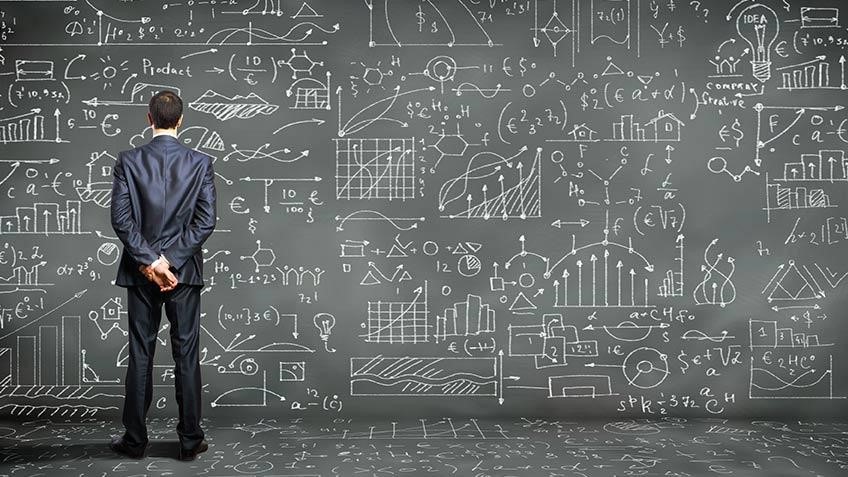
The "new" discipline of Data Science was first coined by the Danish scientist Peter Naur in 1974 in the book Concise Survey of Computer Methods. From the nineties it began to have more and more echo, defining this science as the trilogy formed by the collection of data, its analysis and modeling, and decision making.
At the beginning of the 21st century, the publication of the Data Science Journal and The Journal of Data Science began, journals focused on advances in this field and where professionals began to share their ideas.
In 2012, in an influential article from Harvard Business Review it was published that data scientist was the most "sexy" profession in the world and in 2014 the first IEEE International Conference on Data Science and Advance Analytics was launched.
If we try to define the people who work as Data Scientists they would be a mix between statisticians, computer engineers and creative thinkers. The process performed by a Data Scientist can be summarized in the following 5 steps:
- Extract the data, regardless of where it comes from and its volume.
- Clean it, removing the distortions.
- Process it using different statistical and mathematical methods.
- Design new experiments if necessary.
- Visualize the data graphically.
Being this a general definition of their tasks, what about the professions that already have such skills, but which we deny are Data Scientists? Are they "old" Data Scientists? Let's see some examples of such serious professions.
Actuary
An actuary is an actuarial science professional who deals with the financial implications of risk and uncertainty. These professionals must have solid knowledge in mathematics, economics, finance, demography, probability and statistics.
In addition, they must have knowledge in programming to implement mathematical models for decision making and simulation of risks.
Quant
A quantitative analyst or quant is a professional who designs and implements complex mathematical models that allow financial firms to operate with investment securities.
Normally they must have great knowledge in mathematical concepts, such as calculus, algebra, differential equations, probability and statistics. Also in finance, such as portfolio theory, risk products and derivatives of interest and capital.
In addition, software skills are critical, using Monte Carlo techniques and Machine Learning.
Marketing analyst
A marketing analyst is a professional who investigates what factors the client considers when buying a product or service. The results help companies develop new products or modify existing ones.
These professionals must be able to understand and interpret large amounts of data, so they have to have knowledge in statistics, mathematics and computing in order for them to communicate their results, convert the data into tables, graphs and reports.
Other profiles
There are many other professions with the same characteristics, such as meteorologists who create climate prediction models; the developers of critical systems; those who develop complex systems of air traffic control or signaling; the strategic consultants, who design neural networks to model the preferences of their clients...
When I see these jobs, which already existed in the market previously, I think the key question we should ask ourselves is: what does the definition of Data Scientist lack so that it is what we currently understand?
We are talking about using Big Data, the latest trends in Artificial Intelligence, which are capable of collecting IoT data and use the most novel tools of the visualization market.
That is, if we want a Data Scientist who has previously been a Data Engineer to update their technological knowledge constantly and, in order to get the most out of the conclusions, maybe they should also know about business ... are we then looking for a unicorn?
Comments are moderated and will only be visible if they add to the discussion in a constructive way. If you disagree with a point, please, be polite.
Tell us what you think.